On March 28 and 29, KAIST hosted Google DeepMind researcher Joel Z. Leibo’s seminar and talk on multi-agent reinforcement learning. This particular field of artificial intelligence (AI) is known by many to be one of the fastest-advancing forefronts in academia and at the same time one of the most notoriously challenging ones. The KAIST Herald met with Dr. Leibo to discuss a few mind-boggling issues for AI researchers as well as directions and a word of advice for aspiring researchers.
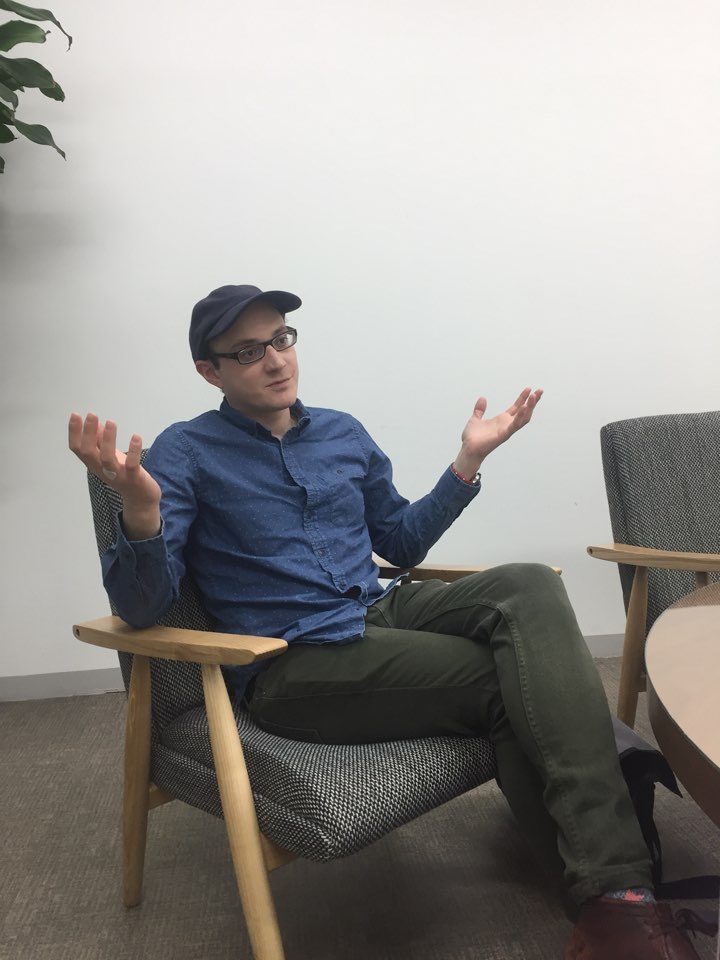
Could you begin by introducing yourself?
My name is Joel Leibo, and I am a researcher with the neuroscience team and the multi-agent team at DeepMind. The goal of DeepMind, as you know, is to build general artificial intelligence.
How far would you say we are into the development of artificial general intelligence?
I think we’ve made a lot of progress recently. We see artificial intelligence employed in technology we use every day, like in our phones. It’s permeating us more and more in general.
What would you say is the single most challenging issue in replicating the success in single-agent reinforcement learning problems to the multi-agent setting? Would you say that the latter is an extension of the former in the first place or that there is something “extra” once we step into the multi-agent setting?
Well, I actually think that the single-agent setting is a special case of the multi-agent setting, where the number of agents equals one. I think that’s actually a better way to see it, with the multi-agent being the more general problem. And if you see it that way, the world looks slightly different. We have made a lot of progress on the specific case where there are fully competitive problems, and we have also made some progress on the case where there are fully cooperative problems, which are in some ways related to the single-agent problem with a single goal. We’re also looking into the possibility that multiple agents may perceive the same goal in different ways. There is a huge open space of problems for multiple agents each acting according to its own goal, with some competitive and some cooperative behaviors mixed.
As machines become more capable of social and competitive tasks, do you anticipate any adverse effects on the power dynamics between humans and machines?
[Laughs] Well, I don’t really think there’s going to be a single “oracle” artificial intelligence that we’re gonna go and bring our problems to and it’s going to tell us the solution. I think it will be multipolar with many different things, with many different levels of intelligence for many different purposes, and it will be integrated into the fabric of how our society works.
AI research (especially deep learning) is sometimes criticized for its weak theoretical foundation. As a contributor to latest AI research progress, what would you say about this criticism?
I don’t think this is a bad thing; I actually think that it’s an exciting thing. I think that, if you’re a theoretically minded researcher in machine learning, it’s a great time to get into deep learning. I mean, the fact is that it does work, so now there’s the question of why it works. Because there are all these important open-ended questions, now would be a great time to get into deep learning. Just because we don’t have a theoretical foundation doesn’t mean we should just throw it away. In fact, hardly ever does the theory come before the practice; think about all the early electronic gadgets.
AI is a big buzzword, and DeepMind is already one of the most wanted destinations of my colleagues. What advice would you give to aspiring AI researchers?
I’d say that the thing to do is to develop a more interdisciplinary background and not just to focus on computer science or any other single subfield. It would probably be useful to have a broader background on neuroscience, cognitive science, or even broader [subjects] like economics. [This is] because all these fields are impacted by artificial intelligence, and are themselves feeding back in insights to artificial intelligence. There’s really a virtual circle to insights from AI impacting these fields and these fields impacting AI again. And, I think the right thing for any individual to do to get into this field is to have a broader background.