A research team led by Professor Junyong Noh from the Graduate School of Culture Technology developed a deep learning-based unsupervised approach for facial retargeting in animation. The paper was accepted to the 28th and 29th Pacific Conference on Computer Graphics and Applications that will take place from October 18 to 21.
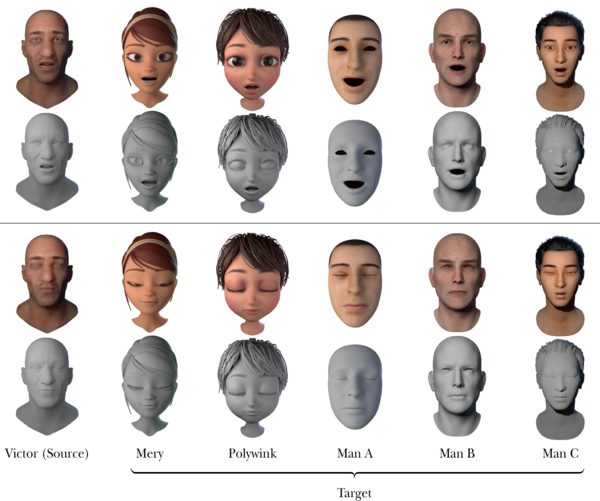
At first glance, creating animated movies and video games might seem to be an enjoyable process. However, any animation artist would agree that their work can also be tedious and overwhelming. In recent years, a great amount of research has been conducted with the aim of automating repetitive tasks in animation to reduce labor costs. One of such tasks is facial retargeting, the process of transferring facial animation from one animated model to another while preserving the semantic meaning of the facial expressions — that is, making animated faces of varying proportions and animation styles that convey the same emotions. The existing facial retargeting approaches require varying amounts of manual work due to their inability to find correct correspondence between faces or to capture the subtle details of facial expressions. Because of these limitations, an artist might have to individually configure facial animations for individual characters.
To tackle this problem, Professor Noh’s team gained inspiration from the recent advances in face reenactment techniques. Similar to facial retargeting, face reenactment refers to the task of transferring facial expressions to a target face, but in the image and video domain. The quality of content produced by reenactment neural networks is astonishingly high, so much so that South Korean channel MBN has recently announced its decision to broadcast face-swapped news anchors for its breaking news reports.
As such, Professor Noh’s team reformulated the facial retargeting task as an image-based face reenactment problem. Inside the retargeting pipeline, a 3D source model is automatically rendered into an image, which is then fed to a reenactment network called ReenactNet. The network outputs the reenacted version of the target image. Lastly, this resulting image is processed by BPNet, a blend shape prediction network, creating the desired facial expression on the target model. This approach enables automatic facial animation retargeting without the tedious process of corresponding facial expression data. Moreover, its results are similar or even superior to the results produced by existing methods, and it is also suitable for both realistic and stylized human characters. Additionally, the pipeline runs in real-time on a consumer-level GPU, meaning that it can be accessible to a wider range of artists.