Professor Sungho Jo from the School of Computing has achieved an improved method in identifying bacteria through the unprecedented fusion of surface-enhanced Raman spectroscopy (SERS) and a newly-devised deep learning algorithm, Dual-branch Wide-Kernel Network (DualWKNet). DualWKNet has proven effective in increasing the accuracy of bacterial classification while reducing time in data collection compared to pre-existing methods.
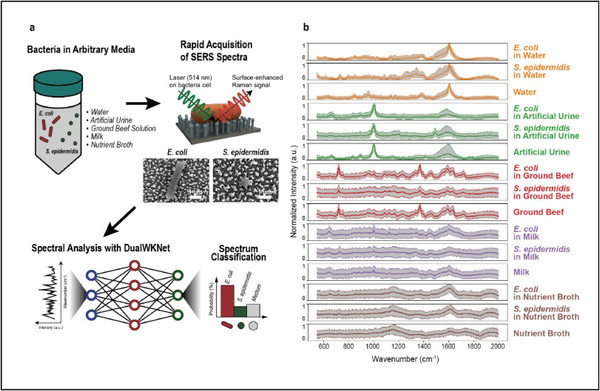
Bacterial identification has far-reaching applications, from diagnosing infectious diseases to testing for food contaminants. Despite the need for faster results, bacterial identification is time-consuming and not accurate enough using conventional methods. Even the most recent techniques such as the polymerase chain reaction (PCR) and enzyme-linked immunosorbent assays require long wait times due to their multi-step processes, while false-negative results are still common for PCR tests.
SERS is a technique that identifies the chemical structure of molecules — including microorganisms. Light is transmitted through a sample placed on noble metal nanostructures, the laser spot pointing at the center of the bacterial cell. The photons scatter depending on the vibration of the molecular bonds present in the cell, producing a spectrum of signals that displays the wavelength and intensity of the scattered light. However, most SERS procedures require the separation of bacteria from its media — surrounding environments that facilitate growth — beforehand. This is because target bacterial signals may overlap with those from their surrounding media, inadvertently causing “noisy spectra” and difficulties distinguishing between the two signals.
Inspired by deep learning used for speech recognition, researchers devised DualWKNet to efficiently identify the “fingerprint” region of the bacterial spectra that provides most of its structural information. This is one of the first instances that deep learning has ever been used for bacterial identification. The dual-branch network of DualWKNet is different from pre-existing models. A single branch includes residual blocks that extract notable spectral features and average pooling layers that prevent information loss by reducing learning parameters. DualWKNet will then predict a class to which the sample belongs by returning probabilities for each class.
DualWKNet was tested on bacteria E. coli and S. epidermidis immersed in different media including artificial urine and ground beef solution to simulate disease diagnosis and food safety tests. Professor Jo remarked, “Despite having interfering signals or noise from the media, which make the general shapes of different bacterial spectra look similar, high classification accuracies of bacterial types and their media were achieved.” Throughout the experiment, DualWKNet could differentiate the key peaks of each bacteria without having to separate them from their resident media beforehand unlike other SERS procedures. DualWKNet showed a superior classification performance of 98% accuracy, at least 3% higher than previous deep learning models such as 1D ResNet and DeepCID.
Professor Jo's research will be published under the title “Separation-free Bacterial Identification in Arbitrary Media via Deep Neural Network-based SERS Analysis” in the April issue of Biosensors and Bioelectronics this year. The research paves way for potential improvements in diagnostic pathology — his team is optimistic as they work towards applying DualWKNet to more bacteria and media types such as blood.