Professor Jaeseung Jeong from the Department of Bio and Brain Engineering worked with a team of researchers to devise a new electrocorticographic (ECoG) decoder used in real-time brain-machine interfaces (BMIs) that translate neural signals to move a robotic arm. The decoder can distinguish between 24 directions of arm movement in three-dimensional space along the x-y, x-z, and y-z planes.
BMIs have significantly enhanced the quality of life for patients with motor dysfunctions caused by Cerebral palsy, Huntington’s disease, and other debilitating disorders. Together with electroencephalography (EEG), ECoG is an established method of recording brain signals through electrodes inserted surgically on the surface of a patient’s cerebral cortex. Compared to EEG, ECoG achieves higher spatial-temporal resolutions and lowers background noises, thus enabling more precise detection of signals. Nevertheless, a limitation is that electrodes can only be placed at specific locations, particularly for patients with brain lesions and epilepsy; thus, not all electrodes can be placed at the optimal areas for sensory and motor processing. The inconsistent position of electrodes at the cerebral cortex across individuals has posed additional setbacks in identifying the neural signals.
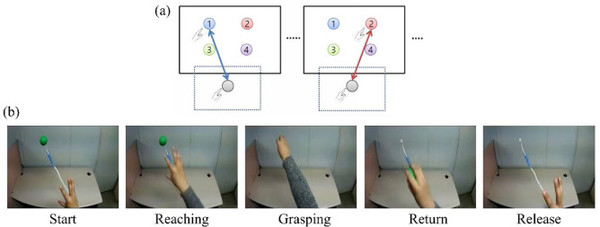
Professor Jeong and his team were motivated to reduce such challenges in current ECoG-BMI studies by designing a new decoder that combines an advanced echo state network (ESN) with Gaussian readouts. The ESN is a machine learning model that analyzes and predicts neural signals via simple linear learning methods, consisting of three layers: input, internal, and readout. Each artificial neuron in the readout (output) layer of the ESN was designed to have preferences for specific directions using Gaussian probability distribution models.
The new ECoG decoder was tested with four patients with intractable epilepsy. With specific numbers of electrodes implanted at different positions in their cerebral cortex, each individual carried out movements of reaching, grasping, returning, and releasing while wearing motion sensors on their wrists and fingers. Some participants were instructed to pick up a real tennis ball presented in front of them, while others watched VR videos of a human arm reaching towards a tennis ball while carrying out the action. Subsequently, the participants imagined repeating the same movements while watching the VR video. The distribution of electrodes for each patient was inconsistent with only 22 to 44% inserted in the motor cortex; however, researchers confirmed that the new ECoG decoder interpreted and differentiated between 24 directions of intended movements in 3D space during both the physical and imagination tasks.
Professor Jeong’s research was published under the title “An Electrocorticographic Decoder for Arm Movement For Brain-Machine Interface Using an Echo State Network and Gaussian Readout” in the March issue of Applied Soft Computing. The research team is looking forward to its increased usage in real-time BMI devices while working toward improving its accuracy and durability. The research stands at the frontier of brain engineering with the hopes of benefiting more individuals with impaired movements and neurological disorders.