Distinguished Professor Sang Yup Lee's research team from the Department of Chemical and Biomolecular Engineering has created a sophisticated artificial intelligence system to predict potential drug interactions. The DeepDDI2 technology developed by the team was able to analyze interactions between Paxlovid, a Pfizer COVID-19 treatment pill, and over 2,200 other prescription drugs. The study was published in the Proceedings of the National Academy of Sciences of America (PNAS) on March 13.
DeepDDI2 is an improved version of DeepDDI, a drug interaction prediction model developed by the same team in 2018. A drug-drug interaction (DDI) refers to the interaction between a drug and another substance that decreases drug efficacy. The new model can process and compute a total of 113 DDI types, as opposed to the previous model's 86 types. The research team used DeepDDI2 to predict potential interactions between ritonavir and nirmatrelvir, the ingredients of Paxlovid, as well as other prescription drugs. They predicted that ritonavir could interact with 1,403 prescription drugs, while nirmatrelvir could interact with 673 drugs.
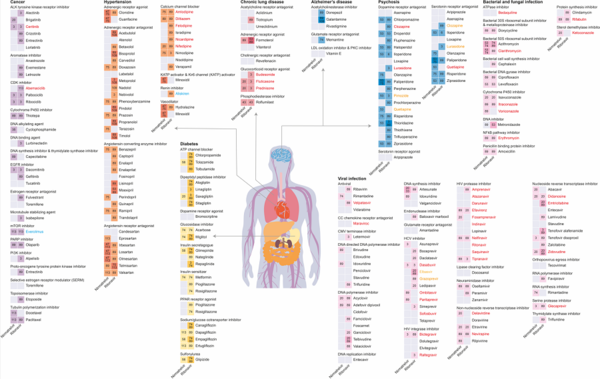
To address the potential risks associated with these drug interactions, the research team proposed alternative drugs to ritonavir and nirmatrelvir that act through the same mechanism but a lower potential for interactions with other prescription drugs that might result in a high risk of adverse drug events. They discovered 124 alternative drugs to ritonavir and 239 to nirmatrelvir that could reduce the possibility of adverse DDI.
The study's findings have significant implications for healthcare and pharmaceutical industries, as they could aid in the development of new drugs and the prescription process. “The results of this study are meaningful when we would have to resort to using drugs that are developed in a hurry in the face of urgent situations like the COVID-19 pandemic. Now it is possible to identify and take necessary actions against adverse drug reactions caused by drug-drug interactions very quickly,” Professor Lee commented.
DeepDDI2 represents a significant advancement in the prediction of drug interactions, particularly in the context of COVID-19 treatments. This technology could help mitigate the risks associated with adverse drug events and improve patient outcomes.