Amidst rapid developments in the field of deep learning, there are emerging demands for new models, which require substantial time and effort to train and reduce errors. The KAIST Herald interviewed Dongmin Park, a PhD student who has recently developed a new data pruning method that reduces the training time of artificial intelligence (AI) models. His paper “Robust Data Pruning under Label Noise via Maximizing Re-labeling Accuracy” on this novel model will be published in Neural Information Processing Systems (NeurIPS) this December.
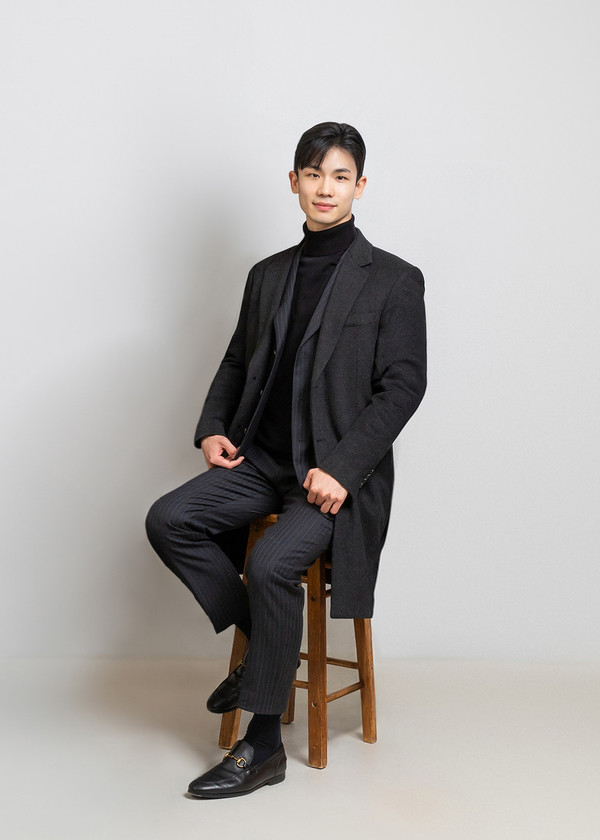
Q. Please introduce yourself to our readers.
Hello, my name is Dongmin Park and I am a 4th year PhD student at KAIST Graduate School of Data Science.
Q. Could you explain your scientific paper?
In the paper, we proposed a new data pruning model that removes redundant or unhelpful data to enhance the performance of the model and reduce the time consumed for training AI models of large real-world datasets. We specifically aim for a “re-labeling” learning method, in which the noise data autonomously adjusts to attain higher performance. Based on the observation that there is a high correlation between the reliability of neighboring data for specific data and re-labeling performance, we proposed this new data-pruning model that prioritizes the significant data that maximizes the sum of the reliability of the neighboring data for the whole dataset. We have proved empirically that this new data-pruning model outperforms the original method in all four real-world noise datasets.
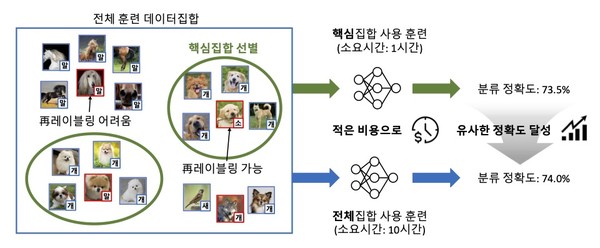
Q. If there were any difficulties in the research, how did you overcome them?
When you conduct research, problems can occur at every single step. This is because research is [the process of] identifying a certain significant issue for the first time and providing a solution for it. The best way to overcome any difficulties is to continuously [discuss and] debate the problem with colleagues.
Q. How can this technology be developed and applied, especially in South Korea and KAIST/scientific research?
There are many applications for this new model of deep learning, especially as it can accelerate the deep learning of the AI model. I hope [our] model can aid your research or any possible applications.