KAIST’s PIM Semiconductor Research Center, led by Professor Hoi-Jun Yoo, developed the world’s first Complementary-Transformer AI semiconductor chip capable of processing a large language model (LLM) at a high performance while minimizing power consumption. The newly developed chip can process GPT-2 with an ultra-low power consumption of 400 milliwatts and a high speed of 0.4 seconds.
This new technology is a type of neuromorphic computing system designed to mimic the structure and function of a human brain. It selectively combines the concepts of Spiking Neural Networks (SNN), which are artificial neural networks that process information using time-based signals called spikes, and Deep Neural Networks (DNN), a deep learning model used for visual data processing. This integrated approach allows for crucial transformer functionalities, essential for learning context and meaning by tracking internal relationships of words in sentences.
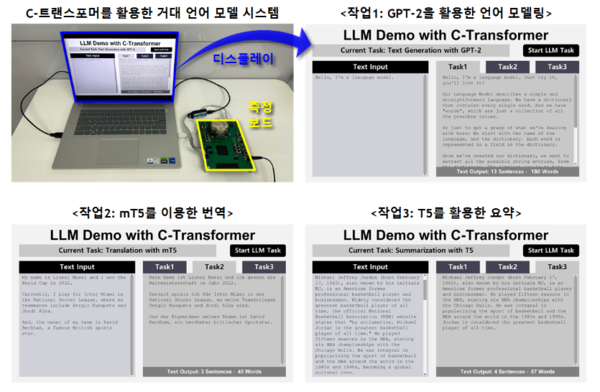
LLMs, such as GPT, normally require multiple graphics processing units (GPUs) and around 250 watts of power consumption. On the contrary, the research team succeeded in operating from a single 4.5mm-square chip with 625 times less power consumption compared with the current AI chip market leader Nvidia's A-100 GPU.
Previous attempts using basic neuromorphic computing technologies were significantly less accurate compared to the standard Convolutional Neural Networks (CNN) and hence were limited to simple image classification tasks. However, the researchers explained that they used Complementary Deep Neural Network (C-DNN) technology, which raises the accuracy of neuromorphic computing technology to the same level as CNN and can be applied to various applications.
The research team not only proved that ultra-low power and high-performance on-device AI is possible, but also implemented the research in the form of AI semiconductors for the first time in the world. The AI semiconductor hardware unit developed through this research has four distinctive features compared to existing large language model semiconductors and neuromorphic computing semiconductors: a unique neural network architecture that combines DNNs and SNNs to optimize computational energy consumption while maintaining accuracy; an integrated core structure for the AI semiconductor that efficiently processes neural network operations; the development of an output spike guess unit to reduce the power consumed in SNN processing; and the use of three compression techniques: a Big-Little Network structure, implicit weight generation, and sign compression to effectively compress the parameters of large language models.
These features reduced the GPT-2 model's parameters from 780 million to 191 million, and the T5 model's parameters used in translation from 402 million to 76 million, respectively. As a result of this compression, it successfully reduced the power consumption to retrieve the language model's parameters from external memory by 70%. Although the accuracy of language generation decreased by a perplexity factor of 1.2, the researchers explained that the level of accuracy did not make the generated sentences awkward.
Looking ahead, the research team plans to expand neuromorphic computing to various applications beyond language models. Professor Yoo expressed his pride in the achievement, stating, "Neuromorphic computing, a technology not yet realized by giants like IBM and Intel, has been successfully implemented in running large models with ultra-low power consumption for the first time worldwide. As a pioneer of on-device AI, we are committed to continuing our research in this promising field.”